Reed Muller Codes in 5G
The Reed Muller codes are used in 5G to encode the small payload of control information having a block length between 3 to 11. These codes are linear block codes which generalizes the Reed–Solomon codes and the Walsh–Hadamard code. These codes are - locally testable, - locally decodable, and - list decodable.
In this tutorial, we will analyze the bit error rate performance of Reed Muller Codes for different link conditions characterized by signal to noise ratio(SNR). We further will demonstrate the variation in performance with block-length. The content of the tutorial is as follows:
Table of content:
Import Libraries
Python Libraries
[1]:
# %matplotlib widget
import matplotlib.pyplot as plt
import os
os.environ["CUDA_VISIBLE_DEVICES"] = "-1"
os.environ['TF_CPP_MIN_LOG_LEVEL'] = '3'
import matplotlib as mpl
from mpl_toolkits.axes_grid1.inset_locator import zoomed_inset_axes
from mpl_toolkits.axes_grid1.inset_locator import mark_inset
import numpy as np
5G Toolkit Libraries
[2]:
from toolkit5G.SymbolMapping import Demapper
from toolkit5G.SymbolMapping import Mapper
from toolkit5G.ChannelCoder import ReedMullerEncoder5G
from toolkit5G.ChannelCoder import ReedMullerDecoder5G
[3]:
# from IPython.display import display, HTML
# display(HTML("<style>.container { width:100% !important; }</style>"))
Mapper and Demapper Parameters
Symbol mapping/Demapping is performed for:
BPSK constellation defined by
constellation_type
which encodes1 bit per contellation symbol defined by
num_bits_per_symbol
.The demapper generates log likelihood ratios configured by
hard_out
using a Log-Map (“app”) decoder defined using
demapping_method
.
[4]:
constellation_type = "bpsk"
num_bits_per_symbol = 1
hard_out = False
demapping_method = "app"
mapper = Mapper(constellation_type, num_bits_per_symbol)
demapper= Demapper(demapping_method, constellation_type, num_bits_per_symbol, hard_out = hard_out)
Simulation Parameters
The simulations parameters are:
K
defines block-length.SNRdB
defines Signal to noise ratio (SNR) in dB.numBatches
defines number of batches to compute average BER.
[5]:
K = np.arange(1,12)
SNRdB = np.linspace(-10,0,5)
SNR = 10**(SNRdB/10)
numBatches = 5000
Simulation
This subsection performs the simulation which involve following steps:
Bits generation of length
k
Reed Muller Encoding
BPSK Symbol Mapping of encoded bits
Passing through the AWGN Channel
Adding noise to BPSK symbols with a given SNR
Symbol De-mapping using Log Map Demapper defined by
"app"
.Generates Log likelihood values as
hard_out
is set toFalse
.
Reed Muller Decoding
BER computation
The flow of the implementation can be understood using folowing diagram:
The bit error rate (BER) is computed for every combination of K \(= \{1,2,3,\dots,11\}\) and SNR \(= \{-10, -8, -6, -4, -2, 0\}\) dB and averaged over \(500000\) batches (monteCarloIterations).
[6]:
BER = np.zeros((K.size, SNR.size))
kIndex = 0
snrIndex = 0
for k in K:
#######################################################
################ Generate UCI Payload #################
#######################################################
bits = np.random.randint(2, size = (numBatches, k))
#######################################################
################ Reed Muller Encoder ##################
#######################################################
codeword = ReedMullerEncoder5G()(bits)
#######################################################
################### Symbol Mapping ####################
#######################################################
symbols = mapper(codeword)
decoder = ReedMullerDecoder5G(k, hard_out)
snrIndex = 0
for snr in SNR:
#######################################################
################ Add Noise at Receiver ################
#######################################################
symbs = symbols + np.sqrt(0.5/snr)*(np.random.standard_normal(size=symbols.shape)+1j*np.random.standard_normal(size=symbols.shape)).astype(np.complex64)
#######################################################
################## Symbol Demapping ###################
#######################################################
llrEst = demapper([symbs, np.float32(1/snr)])
#######################################################
################ Reed Muller Decoder ##################
#######################################################
rxBits = decoder(llrEst)
#######################################################
############## Key Performance Metrics ################
#######################################################
BER[kIndex, snrIndex] = np.mean(np.abs(rxBits-bits))
print("For K="+str(k)+", At SNR(dB): "+str(SNRdB[snrIndex])+" | Bit Error Rate: "+str(BER[kIndex, snrIndex]))
snrIndex = snrIndex + 1
kIndex = kIndex + 1
For K=1, At SNR(dB): -10.0 | Bit Error Rate: 0.2138
For K=1, At SNR(dB): -7.5 | Bit Error Rate: 0.085
For K=1, At SNR(dB): -5.0 | Bit Error Rate: 0.012
For K=1, At SNR(dB): -2.5 | Bit Error Rate: 0.0002
For K=1, At SNR(dB): 0.0 | Bit Error Rate: 0.0
For K=2, At SNR(dB): -10.0 | Bit Error Rate: 0.2157
For K=2, At SNR(dB): -7.5 | Bit Error Rate: 0.0908
For K=2, At SNR(dB): -5.0 | Bit Error Rate: 0.0116
For K=2, At SNR(dB): -2.5 | Bit Error Rate: 0.0005
For K=2, At SNR(dB): 0.0 | Bit Error Rate: 0.0
For K=3, At SNR(dB): -10.0 | Bit Error Rate: 0.2242
For K=3, At SNR(dB): -7.5 | Bit Error Rate: 0.08566666666666667
For K=3, At SNR(dB): -5.0 | Bit Error Rate: 0.0122
For K=3, At SNR(dB): -2.5 | Bit Error Rate: 0.0006
For K=3, At SNR(dB): 0.0 | Bit Error Rate: 0.0
For K=4, At SNR(dB): -10.0 | Bit Error Rate: 0.21985
For K=4, At SNR(dB): -7.5 | Bit Error Rate: 0.0873
For K=4, At SNR(dB): -5.0 | Bit Error Rate: 0.0141
For K=4, At SNR(dB): -2.5 | Bit Error Rate: 0.00025
For K=4, At SNR(dB): 0.0 | Bit Error Rate: 0.0
For K=5, At SNR(dB): -10.0 | Bit Error Rate: 0.21676
For K=5, At SNR(dB): -7.5 | Bit Error Rate: 0.08864
For K=5, At SNR(dB): -5.0 | Bit Error Rate: 0.00996
For K=5, At SNR(dB): -2.5 | Bit Error Rate: 0.0004
For K=5, At SNR(dB): 0.0 | Bit Error Rate: 0.0
For K=6, At SNR(dB): -10.0 | Bit Error Rate: 0.21003333333333332
For K=6, At SNR(dB): -7.5 | Bit Error Rate: 0.08703333333333334
For K=6, At SNR(dB): -5.0 | Bit Error Rate: 0.013533333333333333
For K=6, At SNR(dB): -2.5 | Bit Error Rate: 0.0001
For K=6, At SNR(dB): 0.0 | Bit Error Rate: 0.0
For K=7, At SNR(dB): -10.0 | Bit Error Rate: 0.41942857142857143
For K=7, At SNR(dB): -7.5 | Bit Error Rate: 0.30994285714285713
For K=7, At SNR(dB): -5.0 | Bit Error Rate: 0.12808571428571427
For K=7, At SNR(dB): -2.5 | Bit Error Rate: 0.015
For K=7, At SNR(dB): 0.0 | Bit Error Rate: 0.0005142857142857143
For K=8, At SNR(dB): -10.0 | Bit Error Rate: 0.42665
For K=8, At SNR(dB): -7.5 | Bit Error Rate: 0.308025
For K=8, At SNR(dB): -5.0 | Bit Error Rate: 0.13095
For K=8, At SNR(dB): -2.5 | Bit Error Rate: 0.01465
For K=8, At SNR(dB): 0.0 | Bit Error Rate: 0.0003
For K=9, At SNR(dB): -10.0 | Bit Error Rate: 0.41573333333333334
For K=9, At SNR(dB): -7.5 | Bit Error Rate: 0.31122222222222223
For K=9, At SNR(dB): -5.0 | Bit Error Rate: 0.12635555555555555
For K=9, At SNR(dB): -2.5 | Bit Error Rate: 0.0156
For K=9, At SNR(dB): 0.0 | Bit Error Rate: 0.0006222222222222223
For K=10, At SNR(dB): -10.0 | Bit Error Rate: 0.42352
For K=10, At SNR(dB): -7.5 | Bit Error Rate: 0.31212
For K=10, At SNR(dB): -5.0 | Bit Error Rate: 0.13612
For K=10, At SNR(dB): -2.5 | Bit Error Rate: 0.01516
For K=10, At SNR(dB): 0.0 | Bit Error Rate: 0.0001
For K=11, At SNR(dB): -10.0 | Bit Error Rate: 0.43065454545454546
For K=11, At SNR(dB): -7.5 | Bit Error Rate: 0.3102909090909091
For K=11, At SNR(dB): -5.0 | Bit Error Rate: 0.1298
For K=11, At SNR(dB): -2.5 | Bit Error Rate: 0.015181818181818182
For K=11, At SNR(dB): 0.0 | Bit Error Rate: 0.00021818181818181818
Performance Evaluation
The script plots SNR in dB vs BER performance for block-length \(\in \{1,2,3,\dots,11\}\). It can be observed that the Reed Muller codes can provide the reliablilty of
\(99.99990 \text{ or BER = } 10^{-4}\) for block-length \(\in \{1,2,3,4,5\}\) and,
\(99.99999 \text{ or BER = } 10^{-5}\) for block-length \(\in \{1,2,3,\dots,11\}\) at an SNR value of 0 dB. Furthermore, the larger codeblock-lengths perform poorer compare to the small block-lengths.
Performance Plot
[7]:
fig, ax = plt.subplots()
color_tuple = ['blue', 'orange', 'green', 'red', 'purple', 'brown', 'pink', 'gray', 'olive', 'cyan', 'black']
markcolor_tuple = ['gold', 'navy', 'crimson', 'yellow', 'line', 'springgreen', 'black', 'aqua', 'royalblue', 'red', 'green']
linestyle_tuple = ['-', '--', '-.', ':', 'solid', 'dashed', 'dashdot', 'dotted']
marker_tuple = [".", "o", ">", "2", "8", "s", "p", "*", "P", "X", "D"]
# Make the zoom-in plot:
axins = zoomed_inset_axes(ax, 100, loc="upper right") # zoom = 100
axins2 = zoomed_inset_axes(ax, 100, loc="lower center") # zoom = 100
kIndex = 10
for k in np.flip(K):
ax.semilogy(SNRdB, BER[kIndex], color=color_tuple[kIndex%len(color_tuple)],
linestyle=linestyle_tuple[kIndex%len(linestyle_tuple)], lw = 3,
marker=marker_tuple[kIndex%len(marker_tuple)], markersize = 9, label="K = "+str(k))
axins.semilogy(SNRdB, BER[kIndex], color=color_tuple[kIndex%len(color_tuple)],
linestyle=linestyle_tuple[kIndex%len(linestyle_tuple)],
marker=marker_tuple[kIndex%len(marker_tuple)], markersize = 9, label="K = "+str(k))
axins2.semilogy(SNRdB, BER[kIndex], color=color_tuple[kIndex%len(color_tuple)],
linestyle=linestyle_tuple[kIndex%len(linestyle_tuple)],
marker=marker_tuple[kIndex%len(marker_tuple)], markersize = 9, label="K = "+str(k))
kIndex = kIndex -1
ax.legend(loc="lower left")
ax.set_xlabel("Signal to Noise Ratio (dB)", fontsize = 9)
ax.set_ylabel("Bit Error Rate", fontsize = 9)
ax.set_title("[Reed Muller Codes]: SNR(dB) vs BER for various block-length")
#I want to select the x-range for the zoomed region. I have figured it out suitable values
# by trial and error. How can I pass more elegantly the dates as something like
# select y-range for zoomed region
x1 = -4.99
x2 = -5.01
# select y-range for zoomed region
y1 = 0.1295
y2 = 0.13375
axins.set_xlim(x1, x2)
axins.set_ylim(y1, y2)
plt.xticks(visible=False)
plt.yticks(visible=False)
mark_inset(ax, axins, loc1=2, loc2=3, fc="none", ec="0.5")
# select y-range for zoomed region
x1 = -4.99
x2 = -5.01
# select y-range for zoomed region
y1 = 0.0127
y2 = 0.01325
axins2.set_xlim(x1, x2)
axins2.set_ylim(y1, y2)
plt.xticks(visible=False)
plt.yticks(visible=False)
mark_inset(ax, axins2, loc1=1, loc2=2, fc="none", ec="0.5")
plt.draw()
plt.show()
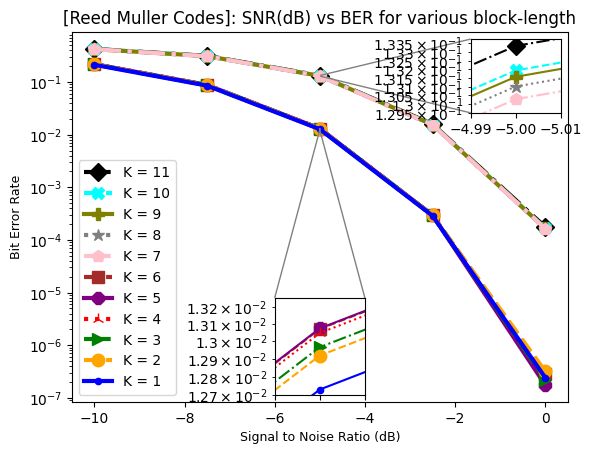
[ ]: