Hamming Codes
Hamming codes are perfect codes for (k,n) when \(k = 2^r-r-1\), \(n = 2^r-1\) where \(r\) is the number of parity bits. The code-rate (\(R = \frac{k}{n} = \frac{2^r-r-1}{2^r-1} = 1 - \frac{r}{2^r-1}\)) of Hamming codes increases as \(r\) increases. Hamming codes can detect \(1\) or \(2\) bit errors and can correct \(1\) bit error.
Table of Content:
Import Libraries
Python Libraries
[1]:
import os
os.environ["CUDA_VISIBLE_DEVICES"] = "-1"
os.environ['TF_CPP_MIN_LOG_LEVEL'] = '3'
import numpy as np
# %matplotlib widget
import matplotlib.pyplot as plt
5G Toolkit Libraries
[2]:
from toolkit5G.SymbolMapping import Demapper
from toolkit5G.SymbolMapping import Mapper
from toolkit5G.ChannelCoder import HammingEncoder
from toolkit5G.ChannelCoder import HammingDecoder
Hamming Codes Parameters
m
is number of parity check bitsk
is number of information bitsn
is size of the codeword
[3]:
## Hamming Code Configurations
m = 3
k = 2**m - m - 1
n = 2**m - 1
Simulation Setup
numBatches
defines numBatches.bits
information bits.encBits
encoded bits.constellation_type
constellation type.num_bits_per_symbol
number of bits per symbols.demapping_method
demapping methods.hard_out
if true symbol demapper return hard bits (0/1) otherwise log likelihood ratios are returned.
Note: For hard outputs, the syndrome based Hamming decoder is used. On the other hand for the soft outputs, the sphere decoder is used.
[4]:
## Payload Generation
numBatches = 10000000
bits = np.random.randint(2, size = (numBatches, k))
## Hamming Encoder
encBits = HammingEncoder(k,n)(bits)
## Rate Matching (No rate matching as of now)
codeword = encBits
## Symbol Mapping
constellation_type = "bpsk"
num_bits_per_symbol = 1
mapperObject = Mapper(constellation_type, num_bits_per_symbol)
symbols = mapperObject(codeword)
SNRdB = np.linspace(-4,8,10)
SNR = 10**(SNRdB/10)
uncodedBER = np.zeros(SNR.shape)
codedBERhard = np.zeros(SNR.shape)
codedBERsoft = np.zeros(SNR.shape)
codedBLERhard = np.zeros(SNR.shape)
codedBLERsoft = np.zeros(SNR.shape)
## Symbol Demapping
demapping_method = "app"
hard_out = False
demapper = Demapper(demapping_method, constellation_type,
num_bits_per_symbol, hard_out = hard_out)
snrIndex = 0
for snr in SNR:
# AWGN Channel
symbs = symbols + np.sqrt(0.5/snr)*(np.random.standard_normal(size=symbols.shape)+1j*np.random.standard_normal(size=symbols.shape)).astype(np.complex64)
# Symbol Demapping
llrEst = demapper([symbs, np.float32(1/snr)])
# Decoding based on Hard Inputs
uncBits = np.where(llrEst > 0, np.int8(1), np.int8(0))
uncodedBER[snrIndex] = np.mean(np.abs(uncBits-encBits))
decoder = HammingDecoder(k,n)
decBits = decoder(uncBits)
# BER and BLER for decoding based on Hard Inputs
codedBERhard[snrIndex] = np.mean(np.abs(bits-decBits))
codedBLERhard[snrIndex]= np.mean(np.where(np.sum(np.abs(bits-decBits), axis=1)>0, True, False))
# Decoding based on Soft Inputs
decoder = HammingDecoder(k,n)
decBits = decoder(llrEst, "sphereDecoding")
# BER and BLER for decoding based on Soft Inputs
codedBERsoft[snrIndex] = np.mean(np.abs(bits-decBits))
codedBLERsoft[snrIndex]= np.mean(np.where(np.sum(np.abs(bits-decBits), axis=1)>0, True, False))
print("At SNR(dB): "+str(SNRdB[snrIndex])+" | coded BER: "+str(codedBERhard[snrIndex])+" | uncoded BER: "+str(uncodedBER[snrIndex]))
snrIndex += 1
At SNR(dB): -4.0 | coded BER: 0.1774301 | uncoded BER: 0.18609175714285714
At SNR(dB): -2.666666666666667 | coded BER: 0.127843825 | uncoded BER: 0.14910897142857144
At SNR(dB): -1.3333333333333335 | coded BER: 0.081641725 | uncoded BER: 0.11257671428571428
At SNR(dB): 0.0 | coded BER: 0.044168875 | uncoded BER: 0.07864995714285715
At SNR(dB): 1.333333333333333 | coded BER: 0.01914105 | uncoded BER: 0.0495807
At SNR(dB): 2.666666666666666 | coded BER: 0.0061892 | uncoded BER: 0.027259314285714285
At SNR(dB): 4.0 | coded BER: 0.001367925 | uncoded BER: 0.012492042857142857
At SNR(dB): 5.333333333333332 | coded BER: 0.000177875 | uncoded BER: 0.004495342857142857
At SNR(dB): 6.666666666666666 | coded BER: 1.205e-05 | uncoded BER: 0.0011498857142857144
At SNR(dB): 8.0 | coded BER: 1.25e-07 | uncoded BER: 0.00019195714285714287
Performance Evaluation: SNR vs BER
Following plots demonstate the BER vs SNR performance of following:
Uncoded transmission (Hamming Code is not used)
Hamming decoder (Syndrome based decoder) taking hard bits (0/1) as inputs.
Hamming decoder (Sphere decoder) taking soft bits (log likelihood ratios) as inputs.
[5]:
fig, ax = plt.subplots()
ax.semilogy(SNRdB, uncodedBER, 'mediumspringgreen', lw = 3, linestyle = "solid",
marker = "*", ms = 12, mec = "crimson", mfc = "darkblue", label = "uncoded BER")
ax.semilogy(SNRdB, codedBERsoft, 'tomato', lw = 3.5, linestyle = (0, (3, 1, 1, 1, 1, 1)),
marker = "s", ms = 6, mec = "k", mfc = "cyan", label = "coded BER [Soft]")
ax.semilogy(SNRdB, codedBERhard, 'k', lw = 3, linestyle = (0, (3, 1, 1, 1, 1, 1)),
marker = "X", ms = 9, mec = "green", mfc = "olive", label = "coded BER [Hard]")
ax.legend()
ax.set_xticks(SNRdB)
ax.grid()
ax.set_ylabel("Bit Error Rate")
ax.set_xlabel("SNR (dB)")
ax.set_title("BER Performance of ("+str(n)+", "+str(k)+") Hamming Codes with BPSK")
plt.show()
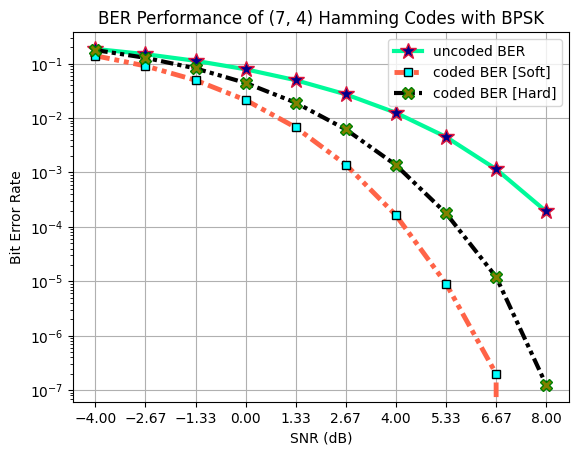
Performance Evaluation: SNR vs BLER
Following plots demonstate the BLER vs SNR performance of following:
Hamming decoder (Syndrome based decoder) taking hard bits (0/1) as inputs.
Hamming decoder (Sphere decoder) taking soft bits (log likelihood ratios) as inputs.
[6]:
fig, ax = plt.subplots()
ax.semilogy(SNRdB, codedBLERsoft, 'tomato', lw = 3.5, linestyle = (0, (3, 1, 1, 1, 1, 1)),
marker = "s", ms = 6, mec = "k", mfc = "cyan", label = "coded BLER [Soft]")
ax.semilogy(SNRdB, codedBLERhard, 'k', lw = 3, linestyle = (0, (3, 1, 1, 1, 1, 1)), marker = "X",
ms = 9, mec = "green", mfc = "olive", label = "coded BLER [Hard]")
ax.legend()
ax.set_xticks(SNRdB)
ax.grid()
ax.set_ylabel("Block Error Rate")
ax.set_xlabel("SNR (dB)")
ax.set_title("BLER Performance of ("+str(n)+", "+str(k)+") Hamming Codes with BPSK")
plt.show()
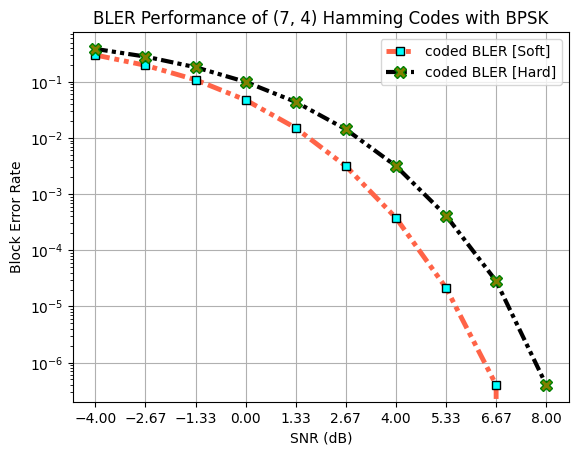
Conclusions
The Hamming decoder with soft inputs outputs the rest of the techniques for both BER as well as BLER.
Sphere Decoding has very high complexity which scales exponentially with parity bits (
m
).
[ ]: